Accelerate IT operations with AI-driven Automation
Automation in IT operations enable agility, resilience, and operational excellence, paving the way for organizations to adapt swiftly to changing environments, deliver superior services, and achieve sustainable success in today's dynamic digital landscape.
Driving Innovation with Next-gen Application Management
Next-generation application management fueled by AIOps is revolutionizing how organizations monitor performance, modernize applications, and manage the entire application lifecycle.
AI-powered Analytics: Transforming Data into Actionable Insights
AIOps and analytics foster a culture of continuous improvement by providing organizations with actionable intelligence to optimize workflows, enhance service quality, and align IT operations with business goals.
In the age of artificial intelligence (AI), data is often hailed as the new oil. However, like crude oil needs refinement before it becomes valuable, raw data must be meticulously prepared to fuel AI systems effectively.
Forrester’s Data And Analytics Survey also emphasizes this point, revealing that 23% respondents expressed concern about data integrity, quality or availability as a challenge for their organization when it comes to using AI. This highlights a critical gap: while many businesses are eager to harness the power of AI, they often struggle with the foundational issue of ensuring their data is reliable and well managed.
Data is the cornerstone of AI success because it serves as the raw material from which machine learning models derive insights, make predictions, and generate recommendations. Yet, despite its significance, achieving and maintaining high-quality data for AI isn’t straightforward. Organizations often face a myriad of challenges in their quest to build AI-ready data.
What is AI-Ready Data?
AI-ready data refers to data that is prepared, structured, and centrally accessible for use in training machine learning models and powering AI systems. For AI to be successful, the data needs to be clean, accessible, and in a format that AI algorithms can easily process.
AI-ready data is not just about having vast amounts of information—it’s about having the right data that can be leveraged to build accurate and reliable AI models. Now, the question is what actually makes data AI-ready?
Here are the qualities that define AI-Ready data:
- Organized and structured in a standardized format.
- Conveniently available from a single, centralized source.
- Secure and private, fully compliant with relevant regulations.
- Regularly refreshed to stay relevant and useful.
- Organized to track user behavior and intentions effectively.
What Makes Data Truly AI-Ready?
For AI to deliver accurate insights and drive meaningful outcomes, it all starts with the data. Hence, having AI-ready data is critical for any business looking to invest in AI as it directly impact the quality of AI models and their ability to function effectively. If the data is poorly prepared or lacks the qualities mentioned above, the AI system can produce inaccurate results, make biased predictions, or even fail entirely.
Here are the key elements that make data truly AI-ready
1. High Data Quality and Consistency
The cornerstone of AI-ready data is its quality. It must be accurate, clean, and consistent across different datasets. This includes ensuring that there are no missing values, duplicates, or erroneous data points.
2. Structured and Standardized Format
For AI to process data effectively, it needs to be organized in a structured format. Structured data is easier to analyze, and standardizing this data ensures that different AI models and systems can work with it seamlessly, minimizing errors caused by format inconsistencies.
3. Rich Metadata for Context and Clarity
Metadata is crucial for providing context to raw data. By including information on how data was generated, where it’s from, and any relationships it may have with other datasets, AI models can better understand and process the information, leading to more accurate and actionable insights.
4. Clear Data Lineage and Provenance
For any organization looking to leverage AI, it is important to understand and track the journey of data from its source to its current state. Clear data lineage helps businesses ensure that the data being used in AI systems is reliable and free from corruption. Provenance helps understand the data’s origins, adding another layer of trust and transparency to it.
5. Strong Governance and Compliance Controls
Compliance with legal regulations and data governance practices is not optional—it’s a necessity. Ensuring that your data is secure, ethically sourced, and compliant with laws is vital for both the success of AI and the protection of privacy and trust.
Why Good Data Is Essential for AI Success
Without precise, consistent, and relevant data, AI systems risk producing incorrect results and misguided recommendations, undermining their value.
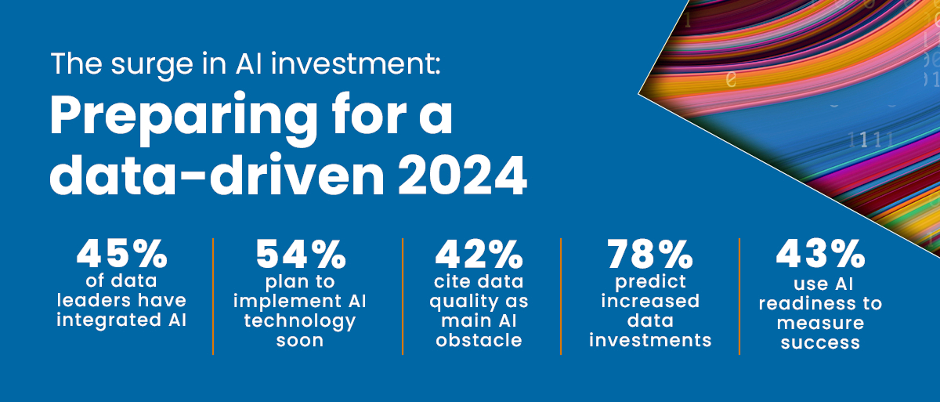
1. Accurate Predictions and Insights
From machine learning models to generative algorithms, AI systems heavily rely on data to deliver accurate predictions and generate valuable insights. According to McKinsey, companies that leverage high-quality data are 2.5 times more likely to outperform their competitors. This statistic highlights how crucial it is for data to be not only abundant but also precise and reliable.
2. Enhanced Decision-Making
Good data enables AI to provide actionable insights that drive better decision-making. High-quality data ensures that AI outputs are dependable and relevant, ultimately guiding more effective business strategies.
3. Building Trust and Adoption
AI systems need stakeholder buy-in to succeed. High-quality data fosters confidence in AI outputs, making it easier for organizations to secure support and integrate AI solutions effectively. Gartner notes that organizations with superior data quality report up to 50% higher returns on their AI investments, underscoring the importance of data in building trust and achieving ROI.
Challenges in Achieving AI-Ready Data
Despite the clear importance of high-quality data, organizations often face several hurdles in their pursuit of AI readiness:
1. Data Silos and Fragmentation
Data is often scattered across various departments, systems, and platforms, leading to silos that hinder data integration and consistency. This fragmentation can result in incomplete or inconsistent datasets that are less useful for AI applications.
2. Data Inaccuracy and Quality Issues
Inaccurate or outdated data can undermine the effectiveness of AI models. Common issues include incorrect data entries, missing values, and inconsistencies. Addressing these issues requires ongoing efforts in data validation and cleansing.
3. Volume and Complexity
Organizations often deal with a mix of structured, semi-structured, and unstructured data, making it challenging to maintain data quality across different formats and sources.
4. Lack of Data Governance
Effective data governance is essential for enterprises to ensure data quality. Many organizations lack robust data governance frameworks, leading to poor data management practices and reduced data integrity.
5. Resource Constraints
Ensuring high-quality data often requires significant resources, including skilled personnel, technology, and time. Many organizations struggle with resource constraints, which can affect their ability to effectively manage and prepare data for AI.
7 Strategies to Achieve AI-Ready Data
Before diving into strategies, it is essential to define what constitutes AI-ready data. AI-ready data is accurate, comprehensive, and structured in a way that facilitates efficient processing and analysis by AI algorithms.
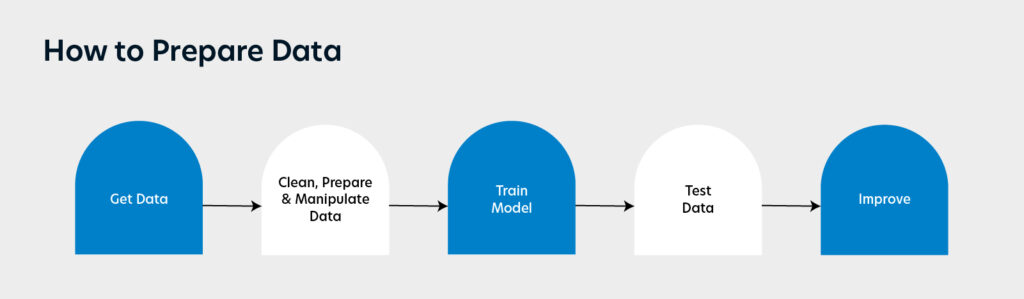
Businesses must ensure that the data they will leverage for training, testing, and deploying AI models has been cleaned, validated, and integrated. Here is what organizations can do to achieve this:
1. Implement a Robust Framework for Data Governance
A framework for solid data governance is the foundation of AI readiness. This framework should include:
- Data Ownership and Accountability: Clearly define who is responsible for managing and maintaining data quality.
- Data Standards and Policies: Establish standards for data entry, storage, and management to ensure consistency and accuracy.
- Data Security and Privacy: Implement policies to protect data from breaches, ensuring compliance with GDPR, CCPA and other regulations.
Effective data governance ensures that data is reliable, secure, and compliant, setting the stage for successful AI implementations.
2. Invest in Data Quality Tools
Data quality tools are essential for maintaining the accuracy and integrity of your data. These tools offer functionalities such as:
- Data Cleansing: Identifying and correcting errors or inconsistencies in data.
- Data Validation: Ensuring data meets predefined quality standards before it is used in AI models.
- Data Enrichment: Enhancing data with additional information to provide more context and improve its value.
According to Gartner, organizations that use data quality tools experience up to a 30% increase in data accuracy, making these tools a worthwhile investment for AI success.
3. Integrate Data across Systems
Data often resides in disparate formats and systems across an organization. Data integration involves consolidating this information into a unified system, which helps:
- Eliminate Data Silos: Break down barriers between different data sources to provide a comprehensive view.
- Ensure Consistency: Maintain data uniformity across various platforms and applications.
- Facilitate Analysis: Streamline data processing for more efficient analysis and AI model training.
Effective data integration is crucial for ensuring that AI systems have access to all relevant information and can perform optimally.
4. Establish Regular Data Audits and Monitoring
Ongoing data audits and monitoring are essential for maintaining data quality over time. Regular audits help:
- Identify Issues: Detect and address data inaccuracies, inconsistencies, or outdated information.
- Track Improvements: Monitor data quality metrics and track the effectiveness of data management practices.
- Ensure Compliance: Verify that data management practices comply with regulatory requirements and organizational standards.
Continuous monitoring and auditing help maintain high-quality data and support the reliability of AI systems.
5. Foster a Data-Centric Culture
Creating a culture that prioritizes data quality and management is crucial for AI success. This involves:
- Educating Employees: Train staff on the importance of data quality and best practices for data management.
- Encouraging Accountability: Promote a culture where all employees understand their role in maintaining data accuracy.
- Promoting Collaboration: Foster collaboration between data management teams and AI practitioners to ensure data meets the needs of AI applications.
A data-centric culture ensures that data quality is consistently prioritized and integrated into daily operations.
6. Utilize Machine Learning for Data Quality
Machine learning algorithms can enhance data quality management by:
- Detecting Anomalies: Identifying unusual patterns or inconsistencies in data.
- Predicting Issues: Forecasting potential data quality problems before they impact AI models.
- Automating Processes: Streamlining data cleansing and validation tasks through automated solutions.
Incorporating machine learning into data quality management can improve efficiency and accuracy, supporting better AI outcomes.
7. Prioritize Data Enrichment
Data enrichment involves enhancing existing data with additional information from external sources. This process helps:
- Improve Context: Provide insights that are more comprehensive by adding relevant external data.
- Enhance Accuracy: Increase the reliability and completeness of data used in AI models.
- Support Better Decision-Making: Offer a more detailed view for more informed decision-making.
Enriched data provides a richer foundation for AI models, leading to more accurate and actionable insights.
Quick Check: Is Your Data AI-Ready?
Meanwhile, to quickly assess whether your data is AI-ready, ask yourself the following questions:
- Is the data clean, accurate, and free from inconsistencies?
- Is it structured in a format that can easily be used by AI models?
- Are you capturing the complete picture of how users interact and behave?
- Are you following data governance standards and complying with relevant regulations?
- Do you have systems in place to keep your data up-to-date and reliable?
If you can answer “yes” to these questions, then your data is likely on the right track to being AI-ready. If not, it might be time to clean up your data and establish processes that will ensure its quality and usability for AI applications.
Conclusion
The journey to AI success begins with a commitment to maintaining and improving the data quality —an investment that pays off in the form of actionable insights, better decision-making, and a competitive edge in the marketplace.
Making data ready for AI success is a multifaceted endeavor that requires strategic planning and execution. By implementing a robust data governance framework, investing in data quality tools, integrating data systems, conducting regular audits, fostering a data-centric culture, leveraging machine learning, and prioritizing data enrichment, businesses can ensure their data is optimized for AI applications.
With AI-ready data, organizations can unlock the full potential of their AI investments, drive innovation, and achieve strategic goals. In the data-driven era, the quality of your data can determine the success of your AI initiatives—so start preparing today for a future powered by intelligent, data-driven insights.
FAQs Related to Making Your Data AI-Ready
AI-ready data refers to data that is properly structured, clean, and formatted in a way that makes it easy for artificial intelligence and machine learning models to process.
AI-readiness is crucial because the quality and structure of your data directly affect how well AI systems perform. Poor-quality or disorganized data can lead to inaccurate predictions, biased outcomes, and unreliable AI models. Having AI-ready data ensures that your systems are built on a solid foundation, improving the accuracy and effectiveness of AI-driven decisions.
To make data AI-ready, start by cleaning it—remove any errors, duplicates, or irrelevant information. Next, structure the data in a consistent format and ensure it’s well-labeled and organized. Enrich the data with metadata to provide context and track its lineage for transparency. Finally, establish governance practices to ensure that the data complies with legal and ethical standards.
Almost every industry can benefit from AI-ready data, but some that see particularly high impact include healthcare (for diagnostics and patient care), finance (for fraud detection and risk assessment), retail (for personalized recommendations), and manufacturing (for predictive maintenance and optimization).
Making your data AI-ready is important because it sets the stage for successful AI implementation. High-quality, well-structured data allows AI models to function optimally, reducing errors and improving the reliability of insights. Without AI-ready data, your AI systems may fail to deliver the results you’re expecting, or worse, produce misleading or biased conclusions.