Accelerate IT operations with AI-driven Automation
Automation in IT operations enable agility, resilience, and operational excellence, paving the way for organizations to adapt swiftly to changing environments, deliver superior services, and achieve sustainable success in today's dynamic digital landscape.
Driving Innovation with Next-gen Application Management
Next-generation application management fueled by AIOps is revolutionizing how organizations monitor performance, modernize applications, and manage the entire application lifecycle.
AI-powered Analytics: Transforming Data into Actionable Insights
AIOps and analytics foster a culture of continuous improvement by providing organizations with actionable intelligence to optimize workflows, enhance service quality, and align IT operations with business goals.
Hey AI Leaders, AI is Powerful, But Will It Work for You?
AI is everywhere. You hear about it transforming industries, automating tasks, and driving billion-dollar efficiencies. But let’s be real—AI is not magic. Implementing AI in your business is not just about plugging in a smart model and expecting miracles. It takes careful planning, testing, and validation to see if AI can truly deliver value for your business.
And this is where the real problem is: Many AI projects fail. According to a RAND research, 80% of AI projects never make it past the prototype stage. Why is that? Because companies dive into full-scale AI implementations without testing feasibility, business impact, or whether AI will actually work in their environment. This is where an AI Proof of Concept (PoC) comes in.
Artificial intelligence PoC acts as your insurance policy against wasted AI investments which allows you to validate your AI idea, test feasibility, and measure real business impact—before committing to full-scale deployment.
A recent survey found that 48% of M&A professionals are now using AI in their due diligence processes, a substantial increase from just 20% in 2018, highlighting the growing recognition of AI’s potential to transform M&A practices.
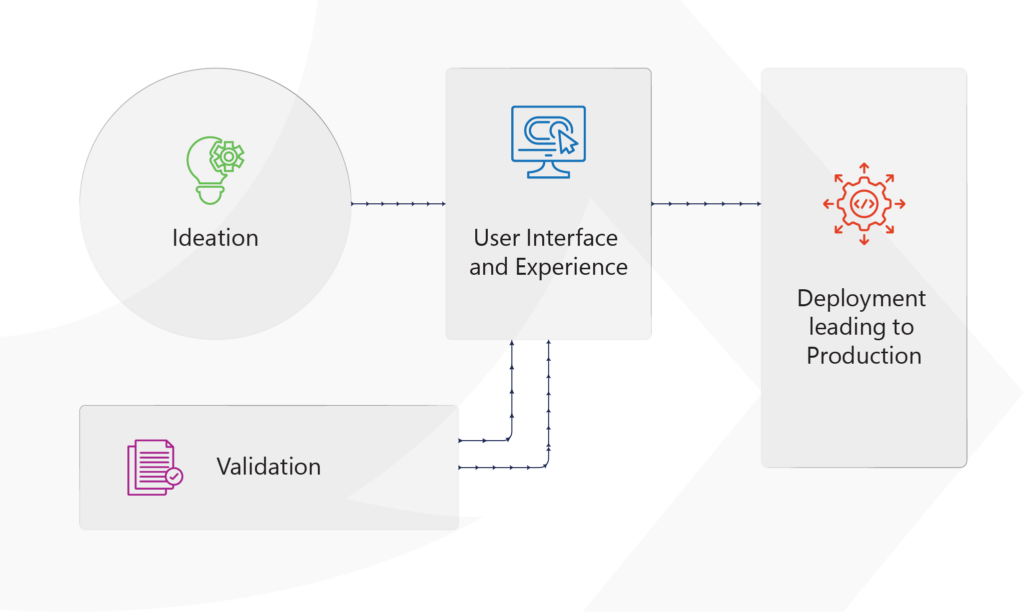
What Is an AI Proof of Concept (PoC)?
An AI Proof of Concept (PoC) is a small-scale, experimental project that helps businesses validate whether an AI solution can achieve specific objectives before full-scale implementation.
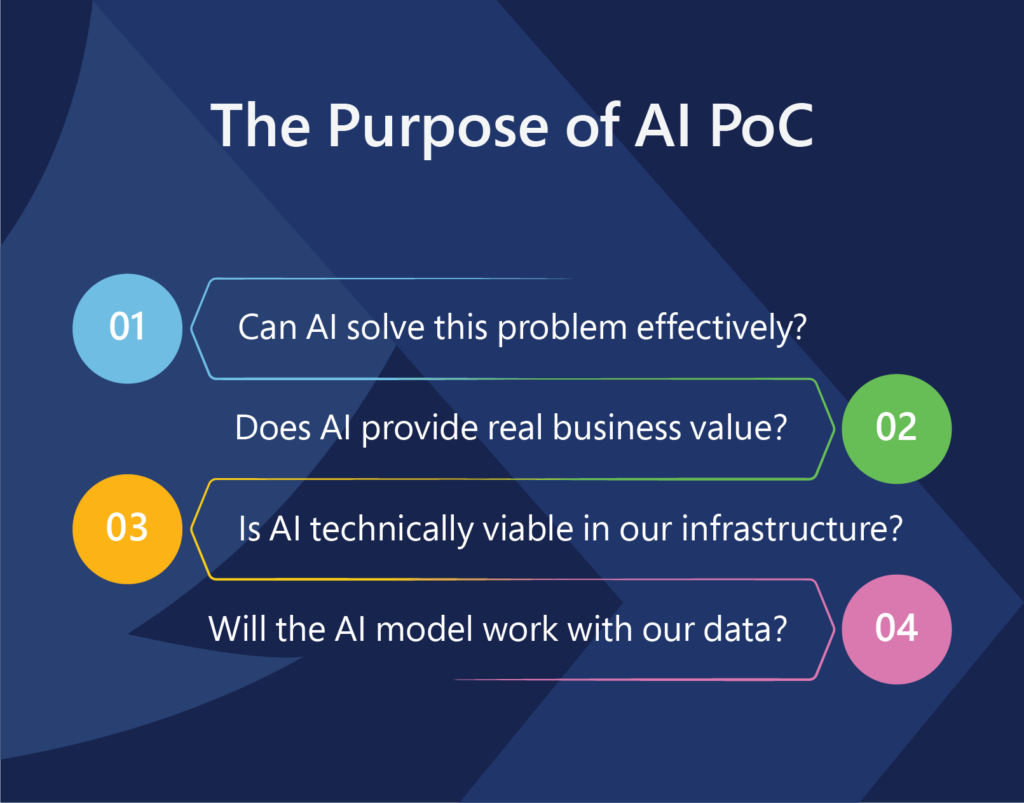
If you don’t have answers to these questions, then you need a PoC artificial intelligence. AI PoC helps organizations gather evidence on the feasibility and impact of AI before making larger investments.
Why AI PoCs Matter?
- AI is powerful, but not all AI projects succeed. The best way to mitigate failure risk is to test feasibility before full-scale deployment.
- AI PoCs helps justify AI investment to stakeholders. Instead of spending millions on untested AI solutions, businesses can validate AI impact in a controlled environment.
- Testing generative AI proof of concept solutions allows companies to explore cutting-edge AI models, ensuring they align with business needs before large-scale investment.
How an AI PoC is different from Prototypes and MVPs
An AI PoC and an AI Prototype are often confused, but all of the three serve different purposes.
|
|
Now, as we know the difference, let’s see when you can consider that your business needs an AI PoC
When Should You Consider an AI PoC (And When Is It a Waste of Time)?
- When AI’s Feasibility Is Uncertain: If your business problem involves large datasets, predictive analytics, or real-time decision-making, but you’re unsure whether AI is the right solution, a PoC can validate AI’s effectiveness before large-scale deployment.
- When No Proven AI Solution Exists in Your Industry: Some industries have mature AI applications, while others (like small-scale manufacturing or logistics) are still exploring AI. If your competitors haven’t deployed AI yet, a PoC helps you experiment and lead the innovation curve.
- When Leadership or Investors Need Proof Before Committing Funds: Executives and investors demand concrete ROI projections before approving AI investments. A PoC delivers measurable evidence of cost savings, efficiency gains, or revenue impact.
- When You Want to Experiment with AI Before Large-Scale Investment: AI is constantly evolving, and businesses may want to explore multiple AI models or vendors before committing. A PoC allows controlled experimentation with different AI solutions.
- When Your Industry Requires AI to Comply with Regulations: In sectors like finance, healthcare, and legal tech, AI must meet compliance and security regulations (GDPR, HIPAA, etc.). A PoC allows businesses to assess AI’s regulatory compliance before a full rollout.
Building AI isn't just about developing smart systems—it's about ensuring those systems are secure, scalable, and built to deliver measurable business value. Without security and strategy, even the smartest AI can become a costly risk.
- Krishna Kumar, VP, Data & AI, Quinnox
When an AI PoC Is a Waste of Time
- If the AI Solution Is Already Proven in Your Industry:
If competitors are already using well-established AI applications, conducting a PoC may be redundant. Instead, businesses should focus on deploying or customizing AI solutions directly. - If You Don’t Have Enough Data to Train an AI Model:
AI models require high-quality, structured data. If your data is incomplete, unstructured, or insufficient, a PoC will yield unreliable results. The priority should be data collection and preparation first. - If Your Organization Lacks AI Talent or Infrastructure:
Building AI requires skilled data scientists, machine learning engineers, and robust IT infrastructure. If you lack these, consider outsourcing AI development or using pre-trained AI models instead of running a PoC. - If a Traditional Solution Can Solve the Problem Efficiently:
AI isn’t always the best solution. If automation, business intelligence tools, or rule-based systems can handle the task more effectively, an AI PoC is unnecessary.
Key Use cases of AI PoC Across Industries
Artificial Intelligence (AI) is transforming businesses by enhancing efficiency, automating processes, and enabling data-driven decision-making. Organizations across industries are leveraging AI Proof of Concepts (PoC) to validate new technologies before full-scale deployment.
Here are 5 real world AI use cases of implementing AI tools with the help of a PoC:
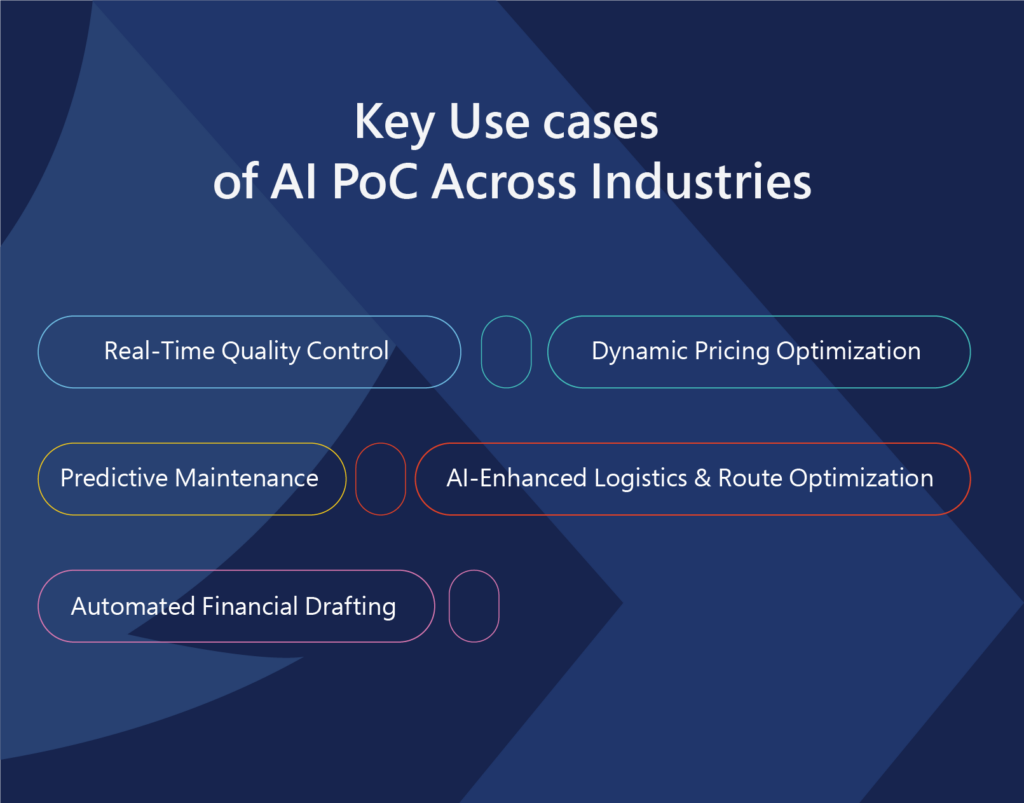
- Real-Time Quality Control – AI-powered image recognition detects defects in manufacturing and retail products, ensuring quality standards are met and reducing human inspection errors.
- Dynamic Pricing Optimization – AI-driven pricing models adjust rates in real-time based on demand, competitor activity, and seasonal trends, maximizing profitability for retailers and service providers.
- Predictive Maintenance – AI sensors track equipment performance, detect anomalies, and predict failures, enabling proactive maintenance to reduce downtime and repair costs.
- AI-Enhanced Logistics & Route Optimization – AI-powered route planning factors in traffic, weather, and delivery priorities to optimize logistics, reducing delays and operational costs.
- Automated Financial Drafting – AI generates financial reports, contract drafts, and regulatory documents by analyzing structured and unstructured financial data, improving accuracy and efficiency.
Sign up to Quinnox AI (QAI) Studio today to get AI POC personalized to your business goals. In addition, get complimentary access to 70+ AI Use Cases here: Quinnox AI (QAI) Studio – Your One-stop AI Innovation Hub
The Biggest Business Benefits that AI PoC Offers
Jumping into AI without validation is a high-risk move—misaligned AI projects can drain resources, fail to meet expectations, and even damage a company’s reputation. An AI Proof of Concept (PoC) acts as a safety net, allowing businesses to test feasibility, measure impact, and refine AI models before committing to full-scale deployment.
1. Early Risk Mitigation:
- 80% of AI projects fail to meet business objectives due to poor planning and misalignment.
- A PoC uncovers technical limitations, data inconsistencies, and business misalignments early on, preventing expensive AI failures at later stages.
2. Smarter Resource Allocation
- A PoC ensures that AI investments—budget, time, and talent—are directed toward proven solutions.
- Instead of blindly committing to AI development, businesses can validate ROI first, ensuring resources aren’t wasted on ineffective models.
3. Defined Success Metrics: Clear KPIs for AI Performance
- Many AI projects fail because businesses don’t define clear success metrics.
- An AI PoC sets measurable KPIs—accuracy, efficiency, cost reduction—ensuring AI aligns with business goals.
4. Faster AI Experimentation & Iteration
- AI requires continuous testing and refinement. A PoC allows businesses to experiment with different models, data inputs, and configurations in a controlled environment before scaling.
- Faster iteration cycles mean businesses can quickly refine AI models and optimize outcomes.
5. Innovation Without Full Commitment
- A PoC allows businesses to explore cutting-edge AI technologies without committing to full-scale implementation.
- This low-risk environment fosters AI innovation while ensuring that only the most promising solutions move forward.
How to Build an AI Proof of Concept?
With iAM, every application becomes a node within a larger, interconnected system. The “intelligent” part isn’t merely about using AI to automate processes but about leveraging data insights to understand, predict, and improve the entire ecosystem’s functionality.
Consider the practical applications:
Step 1: Define the Business Problem & Objectives
Before developing an AI PoC, it is essential to clearly define the problem statement and expected outcomes. This step involves:
- Identifying the challenge – What business problem will AI solve? (e.g., improving customer engagement, optimizing logistics, detecting fraud).
- Defining PoC goals – What will the PoC achieve? (e.g., improve accuracy by 20%, reduce processing time by 50%).
- Setting measurable KPIs – Success metrics should be quantifiable (e.g., response time, cost reduction, accuracy, efficiency gains).
- Understanding constraints – What are the available data sources, budget, infrastructure, and timeline?
Align these objectives with your broader business strategy and ROI expectations. Consider how the AI PoC can contribute to long-term value, whether through cost savings, revenue growth, or improved operational efficiency.
Step 2: Identify the Right AI Use Case
Selecting the right use case is crucial to ensure your AI PoC delivers meaningful results. Evaluate which AI solutions align best with your defined business problem. For instance, customer support challenges can be addressed with chatbots, while sales forecasting may require predictive analytics.
Consider potential ROI when identifying use cases. Solutions that improve customer retention, automate manual processes, or reduce operational costs often provide significant returns.
Evaluate feasibility by assessing:
- Data Availability: Does your organization have sufficient, quality data for model training?
- Workforce Skills: Does your team have expertise in data science and AI development?
- Infrastructure Readiness: Are suitable resources available for computation, storage, and deployment?
Step 3: Choose the Right AI Model (Buy, Build, or Use Pre-Trained Models?)
- Pre-Trained AI Models: These models are ready to deploy for common tasks like image recognition, sentiment analysis, or anomaly detection.
- Pros: Fast implementation, cost-effective, minimal expertise required.
- Cons: Limited customization may struggle with unique use cases.
- Fine-Tuned AI Models: Adapt pre-trained models to your specific business data by retraining select layers.
- Pros: Balanced between speed and customization; leverages existing model strengths.
- Cons: Requires domain expertise and robust data pipelines.
- Ready-Made AI Software: These solutions are ideal for businesses seeking plug-and-play tools with minimal development effort.
- Pros: Simple deployment, low technical expertise required.
- Cons: May not fully meet custom business needs.
- Outsourcing to AI Experts: Partnering with specialized AI vendors can accelerate development and ensure tailored solutions.
- Pros: Leverages expert knowledge, ensuring optimal results.
- Cons: Higher costs and potential dependency on third-party vendors.
Step 4: Collect & Prepare Data for AI PoC
AI models rely on high-quality data for training and validation. In this step, the focus is on:
- Identifying relevant data sources – Structured (CRM, databases) and unstructured (emails, chat logs, images) data.
- Data cleansing & preprocessing – Handling missing values, removing duplicates, and ensuring consistency.
- Feature selection & engineering – Identifying key variables that influence model performance.
- Addressing data privacy & security – Ensuring compliance with GDPR, HIPAA, or other regulations.
Step 5: Develop & Train the AI Model for PoC Testing
Based on your chosen AI model type, follow these development strategies:
- Custom Model Development: Build models using frameworks like PyTorch, TensorFlow, or Scikit-Learn. Custom development offers the highest flexibility for complex use cases.
- Fine-Tuning an AI Model: Enhance pre-trained models using transfer learning. This method is effective for domain-specific applications like medical diagnosis or fraud detection.
- Pre-Trained Model Integration: For simpler AI PoCs, integrate established models into your business environment using APIs.
Throughout development, ensure continuous evaluation of model performance using metrics such as precision, recall, and F1-score.
Step 6: Run AI Experiments & Evaluate Performance
Rigorous testing is essential to validate your PoC’s effectiveness. Simulate real-world conditions by introducing variables such as peak traffic, unexpected data inputs, or complex decision scenarios.
Evaluate model performance across critical metrics:
- Accuracy: Measures prediction correctness.
- Speed: Assesses response time for real-time applications.
- Cost Efficiency: Estimates the financial impact of your solution.
- Scalability: Tests model performance under increased data loads.
Utilize A/B testing and gather end-user feedback to identify areas for improvement before full deployment.
Step 7: Analyze AI PoC Results & Measure Success
Once testing is complete, compare outcomes against your initial KPIs. Identify performance gaps and improvement areas.
Conduct a thorough evaluation of:
- Bottlenecks: Determine if data quality, model architecture, or computational limitations affect performance.
- Failure Points: Understand why specific scenarios failed to deliver expected results.
- Stress Testing: Ensure the model maintains stability under peak conditions.
Step 8: Decide on the Next Step - 4 Real Possibilities!
Based on PoC results, determine the appropriate course of action:
- Full-Scale Deployment: Proceed if KPIs are met and ROI is clear.
- Model Refinement: Enhance data quality, model architecture, or performance tuning.
- Alternative AI Approach: Consider shifting to a different AI method if initial outcomes are insufficient.
- Project Cancellation: If ROI is unachievable or risks are significant, abandon the project and reallocate resources.
Step 9: Transitioning AI PoC to Full Deployment
For successful deployment, create a roadmap that outlines:
- Infrastructure Scaling: Ensure your IT environment can support the AI model in production.
- Security & Compliance: Implement data protection measures and adhere to regulatory requirements.
- Monitoring & Maintenance: Establish systems to track model performance, detect drift, and enable rapid updates.
- Change Management: Provide training for staff to integrate AI capabilities seamlessly into existing workflows.
Common Challenges of AI Development at the PoC Stage
AI development at the PoC stage is often complex, with several obstacles that can delay progress or compromise outcomes. Successfully navigating these challenges requires proactive planning and strategic execution. Here are some common issues organizations face during AI PoC development and ways to address them:
- Inconsistent Data Ecosystem: Data inconsistency, duplication, and outdated information often hinder AI PoCs. Instead of relying solely on historical data, ensure you establish a robust data pipeline that continuously refreshes and consolidates data from multiple sources. Leveraging data versioning tools can further streamline this process.
- Lack of Domain-Specific Knowledge: Even with strong technical expertise, AI teams may struggle to interpret business-specific nuances. Bridging this gap requires collaboration with domain experts who can guide data labeling, feature selection, and model evaluation to ensure the solution aligns with real-world needs.
Related Read: A leader’s guide to bridging AI skills gap
- Resource Optimization Challenges: AI PoCs often demand significant computing power, yet over-provisioning resources can inflate costs. To address this, implement resource-efficient frameworks like lightweight models, transfer learning, or cloud-based infrastructure with auto-scaling capabilities.
- Unclear Evaluation Frameworks: Organizations sometimes struggle to define meaningful success benchmarks during PoC evaluation. Instead of relying solely on model performance metrics, align KPIs with specific business outcomes such as improved customer retention, faster decision-making, or reduced downtime.
- Stakeholder Misalignment: Different stakeholders may have conflicting priorities for an AI PoC. To manage expectations, establish a clear communication framework that defines project goals, milestones, and success criteria upfront. Regular updates and demonstration of early wins can build confidence and alignment.
An AI PoC is more than just a technical experiment—it’s a strategic investment. At QAI Studio, we align every PoC with clear business goals to ensure meaningful outcomes.
– Krishna Kumar, VP, Data & AI, Quinnox
Real-World Success Stories of AI Proof of Concept That You Must See
Case 1: A leading global fragrance company struggled with manually analyzing fragrance formulation requests, limiting response rates to 30-40% of incoming project briefs. QAI Studio developed a Gen-AI-based recommendation engine using LLMs and RAG techniques on Azure, automating formulation analysis. And the results? A 60-70% increase in processed project briefs and ensured real-time regulatory compliance.
See how Quinnox built a Gen AI-powered fragrance formulation engine.
Case 2: A leading UK-based financial services provider specializing in lending and savings solutions, faced challenges in their month-end reconciliation processes. These processes were time-consuming, manual, and prone to errors, causing delays and issues with downstream applications. QAI Studio transformed their month-end reconciliation process by automating key workflows, creating a risk model, establishing control checks, and standardizing processes resulting in reduced processing time by 40%, improved batch performance from 24 minutes to 6 and reduced manual intervention by 80%
See how Quinnox streamlined processes for a financial services firm
The Bottom Line
AI is no longer a futuristic ambition—it is an operational necessity. Whether an organization is at the beginning of its AI journey or scaling its AI initiatives, QAI Studio provides the right combination of tools, platforms, and expertise to drive measurable business impact.
Guru Kandarpi, Head of Global Service Lines at Quinnox.
An AI PoC is your safety net—helping you validate ideas, test feasibility, and measure impact before committing to full-scale deployment. By starting small, businesses can reduce risks, align AI with strategic goals, and unlock real value. Test smart, scale confidently. Start Your journey with Quinnox AI (QAI) Studio today!
In the Infinite Game of application management, you can’t rely on tools designed for finite goals. You need a platform that understands the ongoing nature of application management and compounds value over time. Qinfinite is that platform that has helped businesses achieve some great success numbers as listed below:
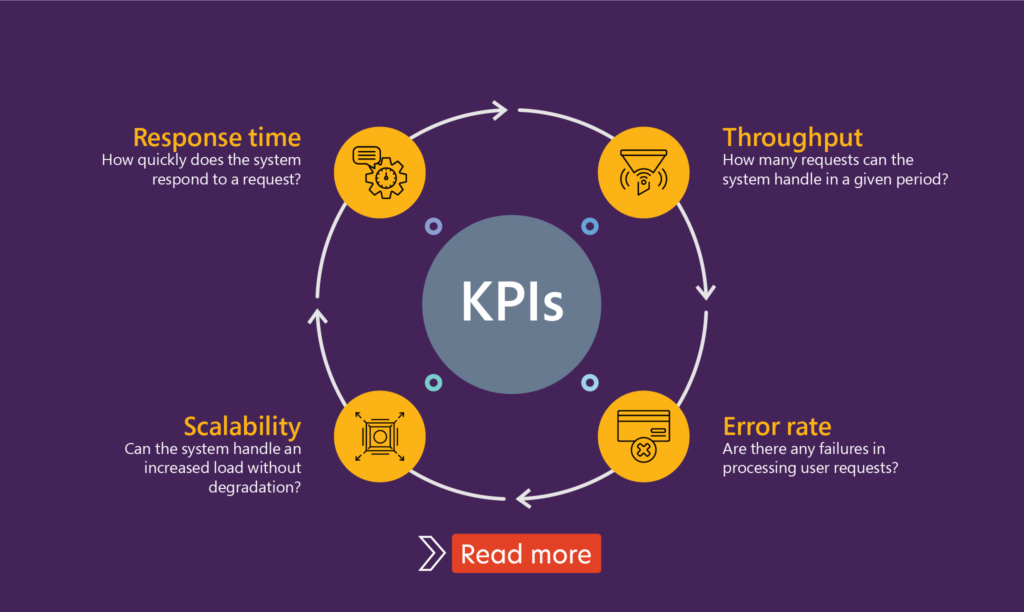
1. Auto Discovery and Topology Mapping:
Qinfinite’s Auto Discovery continuously scans and maps your entire enterprise IT landscape, building a real-time topology of systems, applications, and their dependencies across business and IT domains. This rich understanding of the environment is captured in a Knowledge Graph, which serves as the foundation for making sense of observability data by providing vital context about upstream and downstream impacts.
2. Deep Data Analysis for Actionable Insights:
Qinfinite’s Deep Data Analysis goes beyond simply aggregating observability data. Using sophisticated AI/ML algorithms, it analyzes metrics, logs, traces, and events to detect patterns, anomalies, and correlations. By correlating this telemetry data with the Knowledge Graph, Qinfinite provides actionable insights into how incidents affect not only individual systems but also business outcomes. For example, it can pinpoint how an issue in one microservice may ripple through to other systems or impact critical business services.
3. Intelligent Incident Management: Turning Insights into Actions:
Qinfinite’s Intelligent Incident Management takes observability a step further by converting these actionable insights into automated actions. Once Deep Data Analysis surfaces insights and potential root causes, the platform offers AI-driven recommendations for remediation. But it doesn’t stop there, Qinfinite can automate the entire remediation process. From restarting services to adjusting resource allocations or reconfiguring infrastructure, the platform acts on insights autonomously, reducing the need for manual intervention and significantly speeding up recovery times.
By automating routine incident responses, Qinfinite not only shortens Mean Time to Resolution (MTTR) but also frees up IT teams to focus on strategic tasks, moving from reactive firefighting to proactive system optimization.
Did you know? According to a report by Forrester, companies using cloud-based testing environments have reduced their testing costs by up to 45% while improving test coverage by 30%.
FAQ’s Related to AI POC
An AI PoC is a small-scale project that tests an AI solution’s feasibility and business value before full implementation. It minimizes risks, validates ROI, and ensures AI aligns with business goals.
Consider an AI PoC if you’re unsure about AI’s feasibility, lack proven solutions in your industry, or need to demonstrate ROI to stakeholders before large-scale investment.
Define the business problem, prepare quality data, select the right AI model, develop and train the model, then evaluate results with clear success metrics.
Assess technical performance (e.g., accuracy, precision) and business impact (e.g., cost savings, efficiency gains) while ensuring scalability and user adoption.
Avoid unclear objectives, poor data quality, overlooking success metrics, misalignment with business goals, and skipping stakeholder collaboration.
The choice of AI model depends on the nature of the problem you’re trying to solve. For example, machine learning models are great for predictive analytics, while deep learning models might be used for tasks like image recognition or natural language processing.
While a PoC minimizes risks, success depends on proper execution, data quality, and alignment with business goals.